OpenAI’s decision to make it more straightforward to describe how it presents models shows that there is a continuing problem: not everybody understands what every model is supposed to do and why they are different. OpenAI isn’t alone. If I weren’t reporting on the space daily, I wouldn’t be able to tell the Gemini models apart and tell you why Claude’s Sonnet outshines Haiku. Whereas this confusion is more prevalent with consumers, streamlining products means they are much more accessible, even to power users such as AI engineers. More and more, you need to have a functional encyclopedia in your head of which models can accomplish what and which are more current. Rolling the latest abilities into one model and only permitting more advanced capabilities based on use might make it more accessible for numerous developers to test faster.
The world of artificial intelligence is in fermenting heat, a seething melting pot of technology where new applications and models emerge at a mind-boggling pace. That ferment is even more testament to this revolutionary technology’s dynamism and potential, although it also promises to create a more complex and fragmented environment likely to bewilder users and firms. Wading through this magnum ocean of AI models, which frequently share identical names and ostensibly identical functionalities, is a task that can be intimidating. How to make sense of the various choices? Which model would be most appropriate for one’s particular requirements? The absence of information regarding the precise features of each model, its strengths and limitations, and its true applications generates confusion and frustration, preventing the take-up of AI and restricting its beneficial effects.
Transparency is often lacking
This is paired with the technical excellence of some models, which may appear to be impenetrable ‘black boxes’ to the less experienced users’ gaze. The lack of transparency over the inner workings of these systems, combined with the proliferation of acronyms and technical nomenclature, add to the system being one that is closed off to entry, fueling suspicion and fear towards an otherwise liberating technology that should otherwise be a force in the service of humanity. It is, therefore, essential that AI makers and sellers aim to make their models explainable and accessible to the masses. This involves investing in accurate and thorough documentation that explains the strengths and applications of each model in an easy-to-read and concise form, designing user interfaces that are intuitive and allowing even less skilled users to apply AI software in a natural and spontaneous manner.
More standardization within the terminology and methods used to quantify the performance of AI models would also be appreciated. This would simplify comparison among solutions, enabling users to make more knowledgeable and better-informed decisions, and would promote increased transparency and competition within the industry. However, model-induced confusion is only one of the prominent characteristics of the present AI landscape. Another significant issue is the lack of an adequate public debate regarding the social and ethical consequences of AI.
A more transparent dialogue
AI raises challenging questions regarding privacy, security, work, and what intelligence is. There must be an open, transparent, and honest dialogue among experts, institutions, and citizens to create an ethical and regulatory framework for guiding the development and appropriate utilization of AI so that this technology serves the common good. At some point, AI will significantly transform our world, providing creative solutions for very complex problems and new opportunities in every sphere. But to truly unlock this potential, its challenges of fast change need to be met with clarity and determination. Clarity, transparency, accessibility, and a candid ethical debate are the essential conditions to guarantee that AI is a force for the good of all humanity, building a more equal, fairer, and sustainable future.
Addressing the complexity of AI models is a crucial challenge to make this technology more accessible and understandable. An important first step is the simplification of the models themselves through techniques such as quantization, which reduces the precision of the numbers used; pruning, which eliminates less important connections; and knowledge distillation, which allows smaller models to be trained to mimic complex ones. In addition to simplification, it is crucial to improve the transparency and interpretability of models. Developing explainability techniques, which clarify the AI decision-making process, and using visualization tools to make the inner workings of models more intuitive are key strategies to foster understanding and trust.
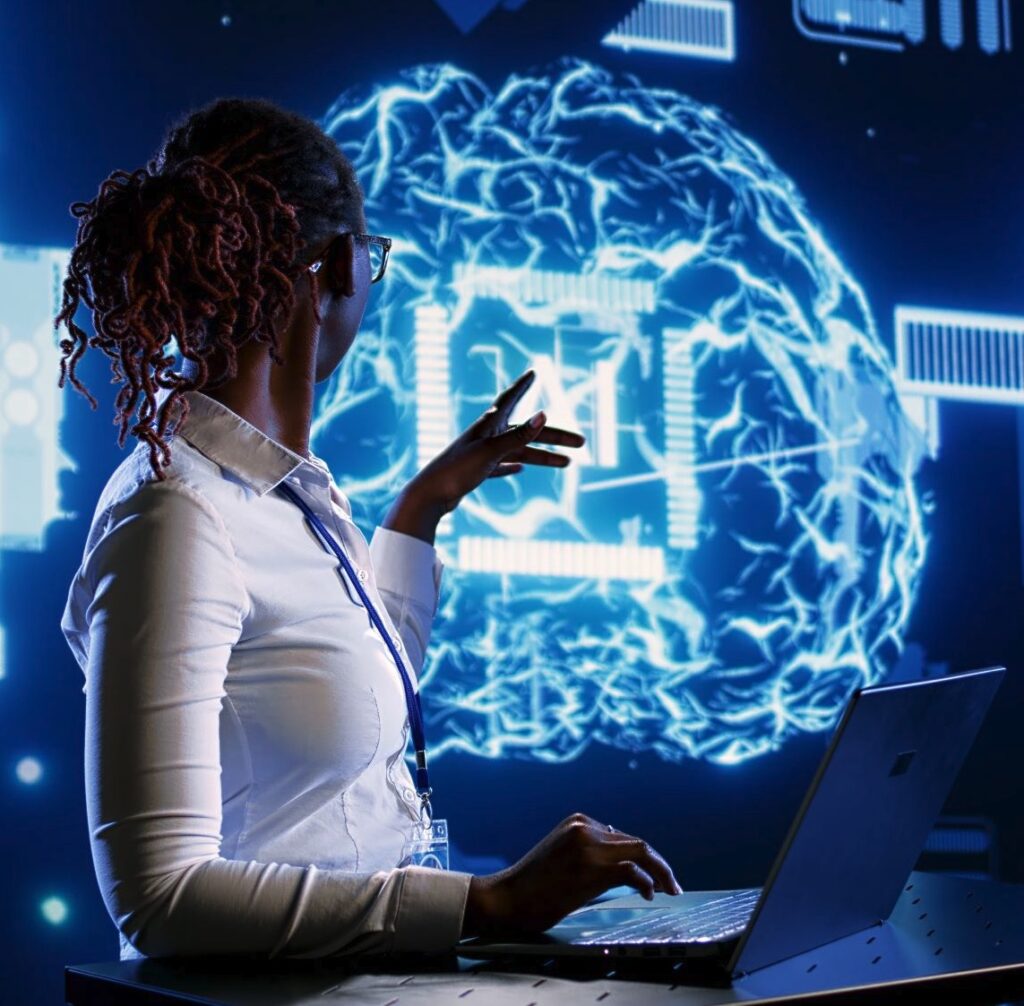
How to reduce complexity
Standardization and modularization play an equally important role. Defining common standards for terminology, evaluation metrics, and model interfaces, as well as developing modular models with reusable components, would simplify design, development, and maintenance and facilitate interoperability and comparison between different solutions. No less important is the role of education and dissemination. Promoting AI education and providing clear and accessible documentation for each model, explaining its functionalities, limitations, and applications, would help spread knowledge and reduce the perception of mystery that often surrounds this technology.
Finally, it is essential to involve end-users in the development process. Gathering and using their feedback to improve the design and usability of models and promoting the co-creation of solutions that respond to real needs are crucial strategies to ensure that AI truly serves people. Reducing the complexity of AI models requires a multifaceted approach involving developers, researchers, institutions and end users. Only through collaboration and joint efforts will it be possible to make AI a more transparent, accessible, and reliable technology that exploits its full potential for the advancement of society.