The new AI, Fake Faces Technology – what does it have in store for the cyber security industry
Technology has reached a point where new algorithms are creating wonderful solutions to different problems that exist in all working fields around the world. Artificial intelligence is a part of that fast and rapid evolution of technology, where every day it aims to generate solutions for different problems in all kinds of fields. Lately AI has been implemented in cyber security industry as well, as a form of problem-solving for different challenges that software engineering companies face every day.
But what can we say about the impact that AI can bring in the cyber world?
It is a fact that AI is bringing change around the world and on our everyday lives, with inventions such as speech recognition technology, Google’s search engine, and Facebook’s facial recognition software etc. Impacts of AI have been massive, where users around the world claim that AI has improved the way of working, has sped up different process, and has made it easier to perform tasks under less time, but with greater quality. But all this wind of change has come with a wave of doubt from different technical experts on how this technology is being implemented, and how it is currently working.
The most common example of an AI algorithm – Facial recognition
One of the most common forms of AI that is being implemented in today’s technology which has a wide spectrum of usage on softwares is considered to be facial recognition. As seen from the cyber security perspective, facial recognition offers a more secure method of authentication when it’s being implemented to different softwares. The facial recognition algorithm can help in reducing risks that exist in different forms of authentications that can be easily exploited, such as cracking passwords, tearing down software firewalls, etc. With the use of facial recognition technology, the security measures are considered to have reached a new level of security, since the use of the humans own face to authenticate over an entity, it is theoretically considered to be an unexploitable form of authentication. But the use of facial recognition has encountered numerous problems along the way, where problems such as BIAS in the way of authenticating and recognizing the human faces have been noticed.
What is AI bias?
AI bias is the underlying prejudice in data that’s used to create AI algorithms, which can ultimately result in discrimination and other social consequences. So to put it in simpler terms, AI bias is a form of discrimination and prejudices towards different social groups that come as a result of lacking data in the making of AI algorithms. AI systems learn to make decisions based on training data, which can include biased human decisions or reflect historical or social inequities, even if sensitive variables such as gender, race, or sexual orientation are removed. But fortunately AI technology is being developed and elevated to a new level, where it can use itself to solve this mishap.
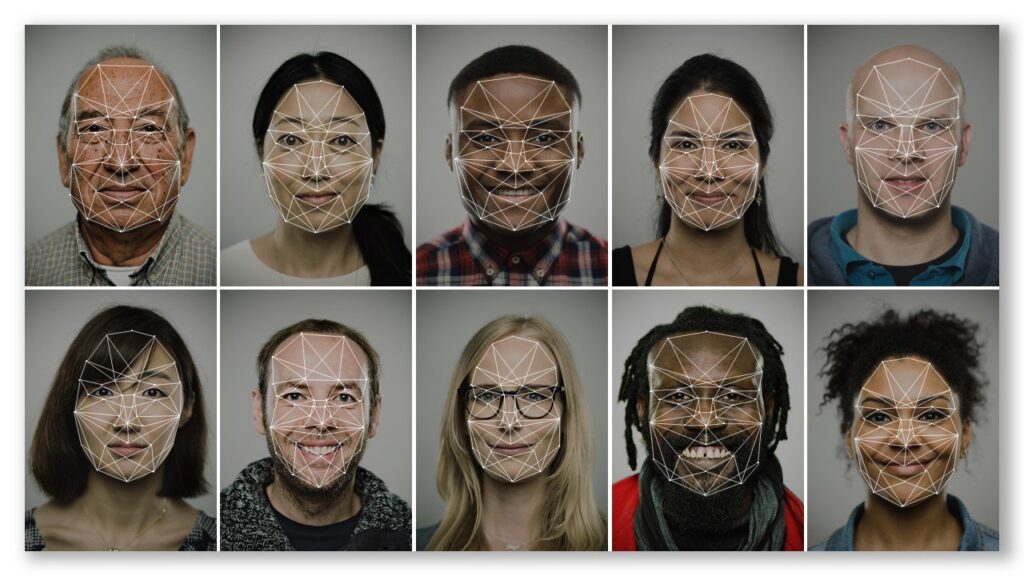
Is GAN the new problem-solving AI algorithm?
A new way on how AI is trying to solve different issues that have surfaced is through GAN technology. The Generative Adversarial Network, or GAN for short, is predicted to be the next big thing in Machine Learning and AI. The core idea of a GAN is to obtain a large set of data, where GAN is capable of generating brand new unique data that is effectively indistinguishable from the original. For a more technical explanation a GAN is a machine learning framework in which two neural networks compete with each other to become more precise in their predictions.
The two neural networks are referred to as the generator and the discriminator. The goal of the generator is to artificially produce outputs that could easily be mistaken for real data. The goal of the discriminator is to identify which outputs it receives have been artificially created.
Basically, GANs create their own training data. Following this model, was how the concept of Fake Faces was created, which is believed to be a new solution on solving AI bias. Basically what happens is that GAN technology gathers a great number of sample data, which in our case are human faces that exist in real life. Then using the GAN algorithm, all these sample data, are used to generate fake faces, that actually don’t exist, but from their appearances they look extremely real and can be easily mistaken for real humans.
A proof concept of Fake Faces AI algorithm can be found on “This Person does’t Exist“, a website created solely to prove that GAN model can generate real life looking faces. The site is a creation of Philip Wang, a software engineer that uses the GAN model to fabricate ‘new faces’ with the help of large datasets that contain real people’s pictures. “Each time you refresh the site, the network will generate a new facial image from scratch,” wrote Wang in a Facebook post. (You can even try it yourself by clicking at the link above).
This solution is thought to solve AI bias once and for all. With the accumulation of real data samples, and also generating fake faces that help in creating large databases, can manage to create an AI algorithm so powerful that can surpass any biases. But this innovative AI model has come along with some concerns from security experts around the world, that believe that the fake faces AI algorithm can create different issues regarding cyber safety.
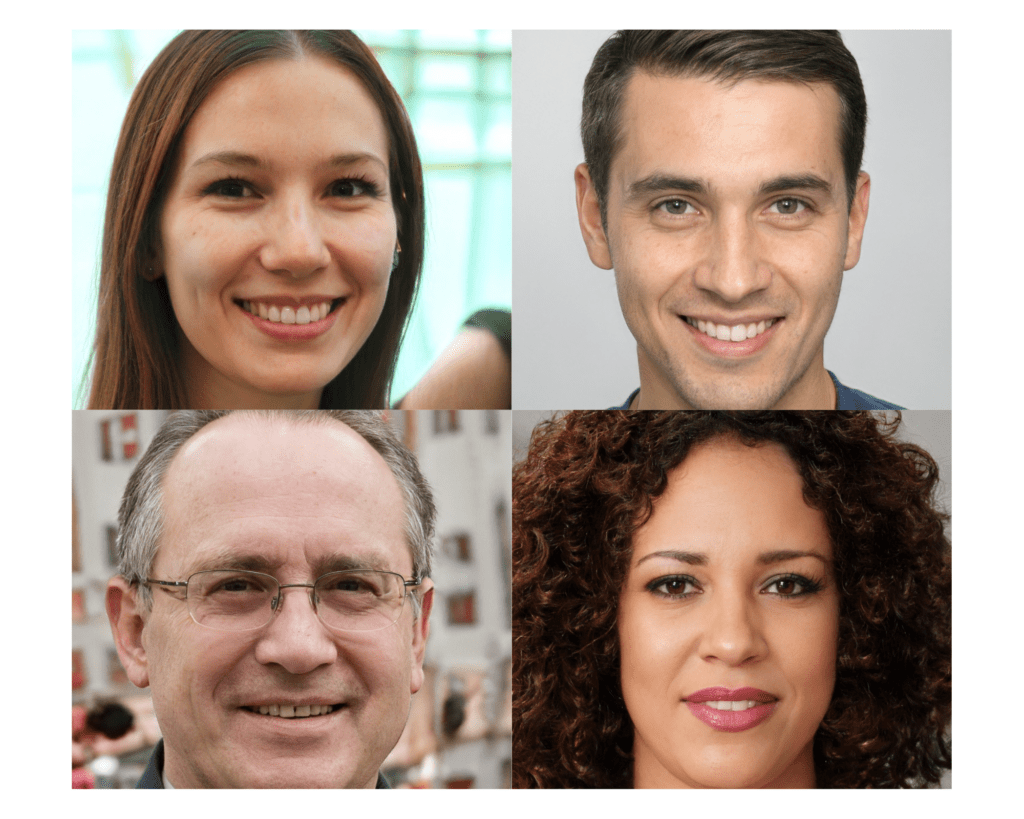
As explained, GAN is a tool that the main purpose of it is generating high quality output that is fake but resembles a lot the original data input. Specifically, a GAN can be used to fool existing image detection systems as well as generating high resolution fake images. This means the common systems in use like the famous Facebook’s Deep Face which claims to have 97% accuracy, can be tricked using adversarial networks.
To sum it up this technology could result in fake images of different public figures, other persons of interest, or even us. This poses a real threat to our personal security, if realistic fake images are being generated massively. And overall what GAN teaches us is that data can’t be always trusted, and it’s is our duty to help in raising awareness of cyber threats that come along undetected with new emerging technologies.